Blog > Data Analytics versus Big Data versus Data Science
Data Analytics versus Big Data versus Data Science
May 2nd, 2022
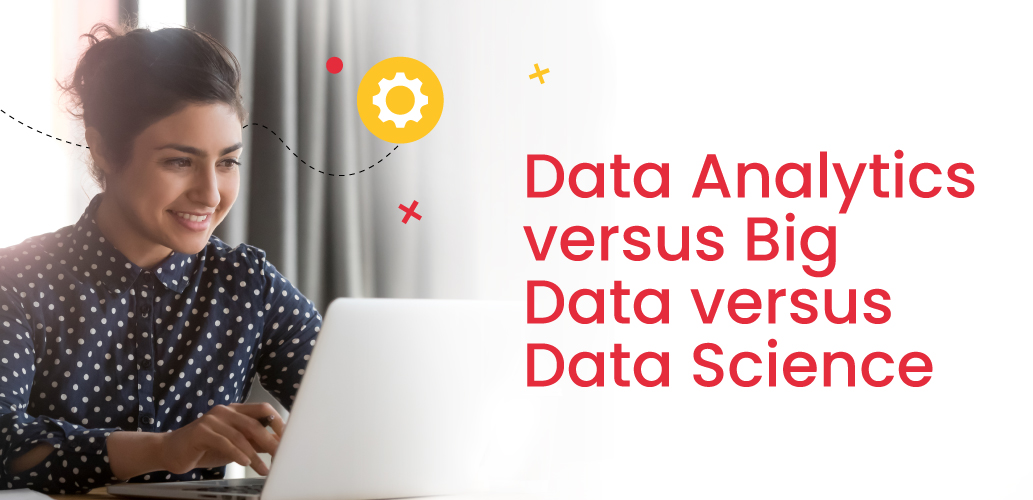
Information is only useful when it’s understood.
This quote has become an emotion in this new era of business, where technology acted as a game-changer. In this day and age where anything can be considered as data and one man’s trash is another man’s gold, how do you navigate your company to higher prospects?
To make data usable it has to first be sifted through and made relevant.
That task is taken up by a talented Analyst.

What is Data Analytics?
Data analytics is an umbrella term that encompasses many different types of analysis. Data can be subjected to various kinds of analytic techniques and tools to refine it and make it useful information. Think of data as crude oil. It’s valuable only because of what it can be converted into. Data analysis is the refinery that separates information from crude data. And just like refineries don’t run themselves, you need specialised people - called data analysts- who are responsible for gaining key insights from the refined information given to them.
Data Analytics can be useful to any industry, it all depends on what the company is looking to improve. Several sectors that have a high turnover, like medical, hospitality, travel, etc, use customer data such as personal information, reviews, complaints, compliments, guest preferences, review forms, etc, to fix or make improvements to existing protocols. Data Analytics in retails helps keep track of the latest trends, bestselling products, and the average spending power of customers, which helps retailers stay afloat in a vastly competitive field. In healthcare, copious amounts of structured and unstructured data, which include past patient records, are refined to make informed and quick decisions.
The goal of Data Analytics in any sector is to make enlightened decisions based on past records, behaviours, patterns, trends, preferences, and any kind of relevant information from the data pool.
So how is Data Analytics any different from Data Science or Big Data?
All three of these terms share certain similarities. They all use the data available around us to improve decision-making and provide key insights for the company.
But the difference emerges in how they derive these insights and what they use to derive them.
To better comprehend how these 3 are different let’s review Big Data and Data science once.
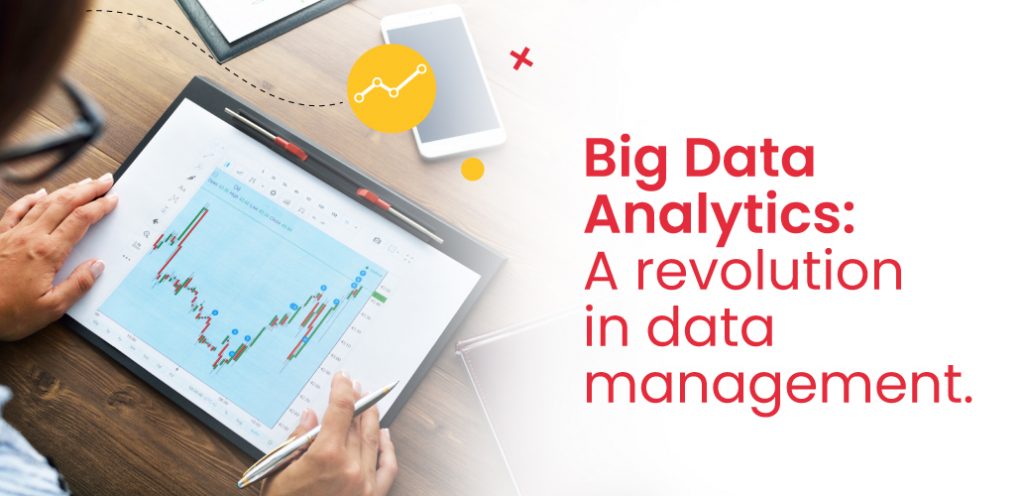
Big Data Analytics: A revolution in data management.
Big Data, just like the name symbolises, deals with data in colossal quantities. Where traditional data sets are mostly in gigabytes or terabytes at most, big data comes in petabytes, zettabytes, or exabytes.
To put this into perspective, if one byte is equal to one metre, then 1GB of data is 1Million kilometres. That is the value traditional datasets work with. 1 petabyte is 1 billion Gigabytes. That is 1000,000,000,000,000,000 metres or bytes. Traditional storage systems are ill-equipped to handle a data set of this size. Big Data is most often stored on the cloud or needs a specialised storage solution depending on where the data is currently residing.
Big Data is differentiated based on these three components:
Volume, as discussed above, means the size of each data set.
Velocity is the speed at which data is derived. The reason Big Data is as big as it is, is because data is constantly generated.
Variety refers to the various sources from which data is collected. Big Data considers data present in texts, comments, likes, etc.
Due to the large volume and various sources of data, Big Data is mostly unstructured in nature and needs a different set of tools to be analysed.
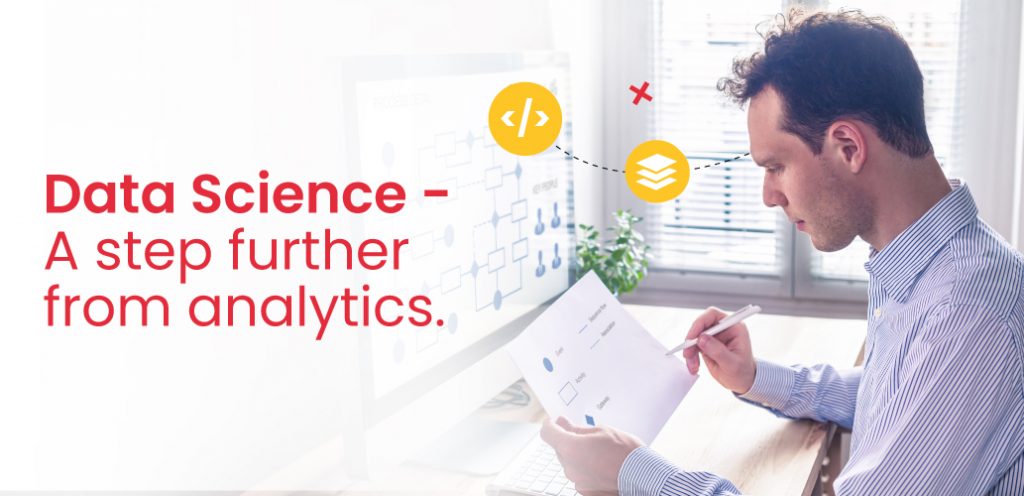
Data Science - A step further from analytics.
Now that we’ve taken cognisance of Big Data and Data Analytics, let’s delve into Data Science. Data science is a multifaceted field, involving extracting information from:
- Scientific methods,
- Maths and statistics
- Programming
- Advanced analytics
- Machine Learning
- Artificial Intelligence
- and Deep Learning
Since the scope of Data Science far exceeds its purpose, i.e, to gain meaningful insights, Data Science deals with analysing complex data, creating new analytics algorithms, tools to further distil the data and even building dynamic visualisations.
Ultimately, there is definitely a degree of truth to the saying data is the new oil.
We have yet to determine the true potential of using data and as we continue our discovery of the subject, the value of data is only going to grow exponentially. This in turn implies that the sectors that discern data are going to become integral to the growth of businesses across continents.
Add a Comment