Blog > How Netflix uses Machine Learning to keep you up till 3 AM.
How Netflix uses Machine Learning to keep you up till 3 AM.
June 8th, 2022
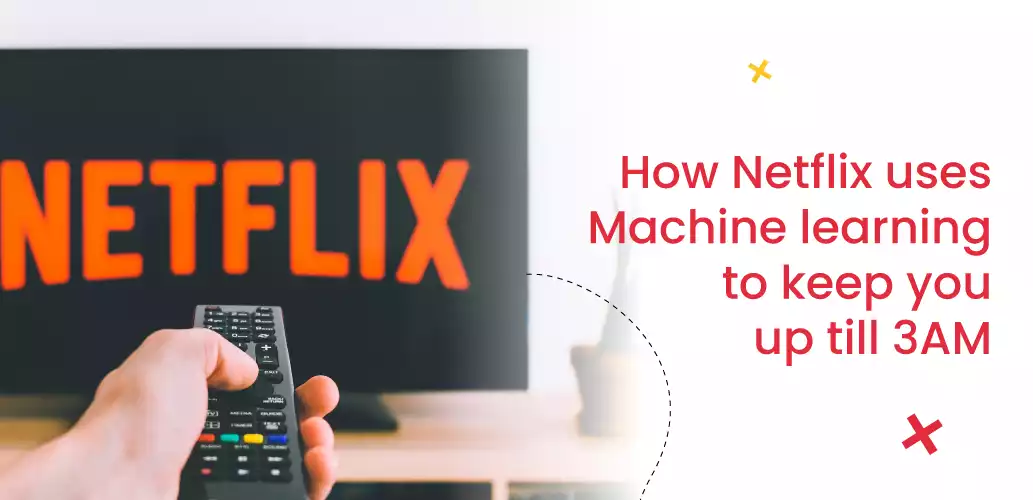
You might not judge a book by its cover, but you definitely watch movies based on your recommendation list. In today’s blog, we’re going to unravel the secret to Netflix’s “bingeability” and why you end up staying awake till 3 in the morning to binge-watch a show you would otherwise never be interested in.
The science behind Netflix "Recommendations"
It’s no secret that Netflix uses Machine Learning and complex algorithms to deliver the best recommendations amongst its competitors.
For those of you still new to the tech scene - an algorithm is a set of database instructions that tell the software or application what to do. Imagine the computer is Dora the Explorer. She needs a map to go about doing new things and adventures. The algorithm serves as a Maps app, the one responsible for charting out the best possible route for Dora to achieve her goals.
In order for Machine Learning to actually be facilitated, the machine needs to obviously learn something. What is that “something”? It’s the data collected from our views, searches and clicks. Every time we watch a movie, search for a title or even click on a movie but not necessarily watch it, our action informs the machine about our possible interests and preferences. The algorithm being extremely sensitive, picks this data and rewrites and adjusts itself, every time we watch Netflix and give it an insight into our tastes.
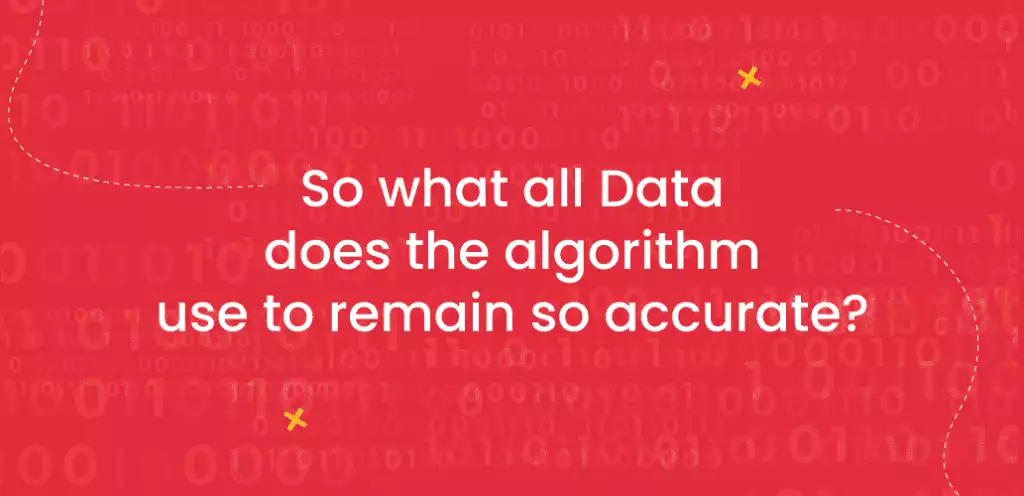
According to Todd Yellin, VP Product at Netflix, the engine takes into account information such as, what people watch, what was it that they watched before and what did they watch after, what they watched a year ago, what all they watched recently and what time of the day did they watch these things.
Netflix can’t just recommend the bestselling movies or the most cinematically advanced films to its viewers. Netflix suggestions have to be based on a viewer’s personality. Instead of just dumping their entire catalogue on a viewer’s home page, they curate lists using different algorithms present in their rankings, search bar, ratings, similarity and more.
An amalgamation of all this information is the driving force behind Netflix’s successful recommendations. It is programmed to accustom itself to the most minor changes you bring to the table. And have you ever noticed how perceptive the suggestions are? You can may have watched one episode of a whole new genre - let’s say an anime or k-drama - but the next thing you know your entire feed slowly starts to change, with suggestions such as “Other K-drama’s you may like”, “Because you watched xyz anime” “The best of East-asia”. And obviously fueled by our own binge-watching beast, we end up watching an entire genre over the course of a month.
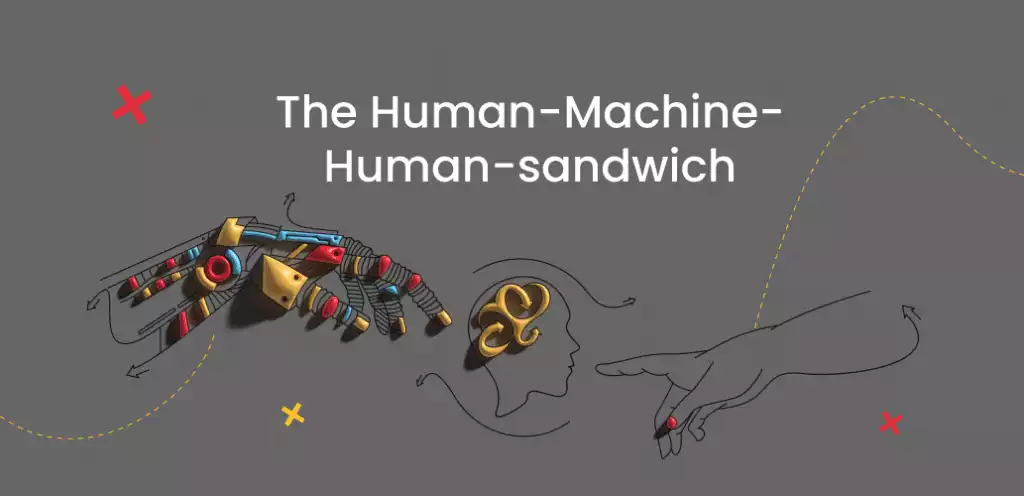
We’ve spoken a lot about the above mentioned algorithm and how presently it tracks our viewing history. However this algorithm wasn’t born ready. And the groups you got grouped into didn’t appear out of thin air. This is the work of actual human beings, brought in to label and group movies into hyper-specific genres like “Visually striking witty comedies” “Classic feel good opposites attract romcoms” or our personal favourite, “Cynical Comedies Featuring a Strong Female Lead”.
Each of these categories is what you get grouped into by the algorithm. And it’s never just one category. Every one of us gets grouped into multiple categories, which then dictate our taste and decide what will appear on our individual home screens.
So without these hyper-specific categories made ready, the algorithm will not be able to complete its main job- analysing data and grouping people into categories.
But that’s not all what Netflix does to rope us into the binge watching cycle.

Netflix figured out that on an average, they had a golden time of 90 seconds. Only 90 seconds. In this one-and-a-half minute, their viewer would make a judgement as to whether or not they were going to watch the movie that caught their attention.
In order for their viewers to evaluate and better understand the content of a film under 90 seconds, Netflix decided to use engaging Movie posters. Neuroscientists have proven that an image can be processed and judged by a human in under 13 milliseconds. Compared to text, which takes a lot longer, an image does speak a thousand words.
The movie posters they put out originally were given to them by the studios at the time, and they were the generic movie posters that would be displayed in cinemas and on billboards. Now while these posters worked for their respective print mediums, Netflix caught on to the fact that it dampened the attractiveness of the movie on their platform for their viewers. Knowing that they had only 90 seconds to appeal to their audience they came up with a series of experiments in order to boost engagement.
They performed a series of A/B tests and explore-exploit tests, through which they tested whether the movie poster shown to the viewer would have an effect on their judgement of the movie itself.
They designed a test that displayed multiple sets of images for each title, where the original movie poster provided by the studio acts as the control in this test. Their results, overall, unanimously proved that the audience/ test subjects reacted more strongly when faced with a complex set of emotions on the posters.
A good example of this test would be Strangers Things, the hit netflix drama series. Notice how many different ways the poster is shown to all the different accounts.
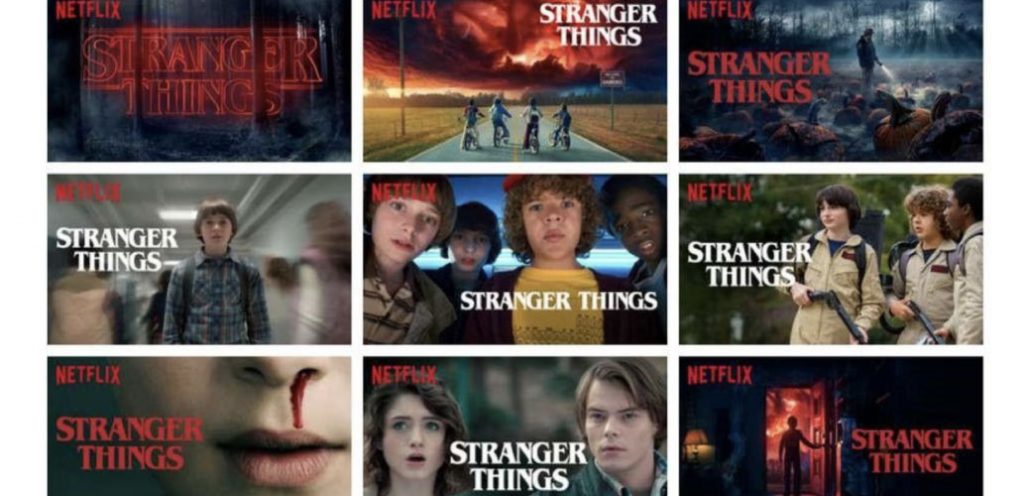
In order to decide which user will be shown which poster, Netflix tracks what the user has been watching again and groups that user into certain categories (again). So consider a movie like “The Intern”. If User X happens to watch more of Anne Hathaway movies as compared to Robert De Niro, they are more likely to click on a movie poster with her face.
The same goes for genres as well. If User Y watches a lot of horror movies, they will react more strongly to a poster that depicts the horror elements of that movie.
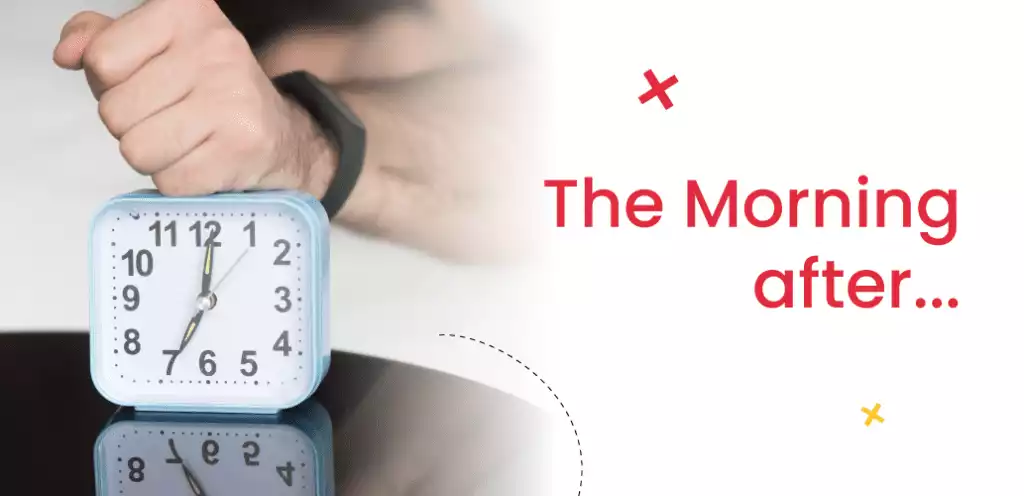
So there you have it - the reason behind all your late night binge watching sessions. It’s a combination of machine learning, human intervention and personalised artwork that have resulted in Netflix’s 1 billion dollar algorithm for recommendations. This award winning strategy, however, is just the beginning to Netflix’s ploy to boost engagement. Don’t get too curious though, since that probably means we’ll just have to pull through more all nighters.
Add a Comment