Blog > Predictive Analytics vs. Financial Modelling: A Comparative Analysis
Predictive Analytics vs. Financial Modelling: A Comparative Analysis
December 28th, 2023
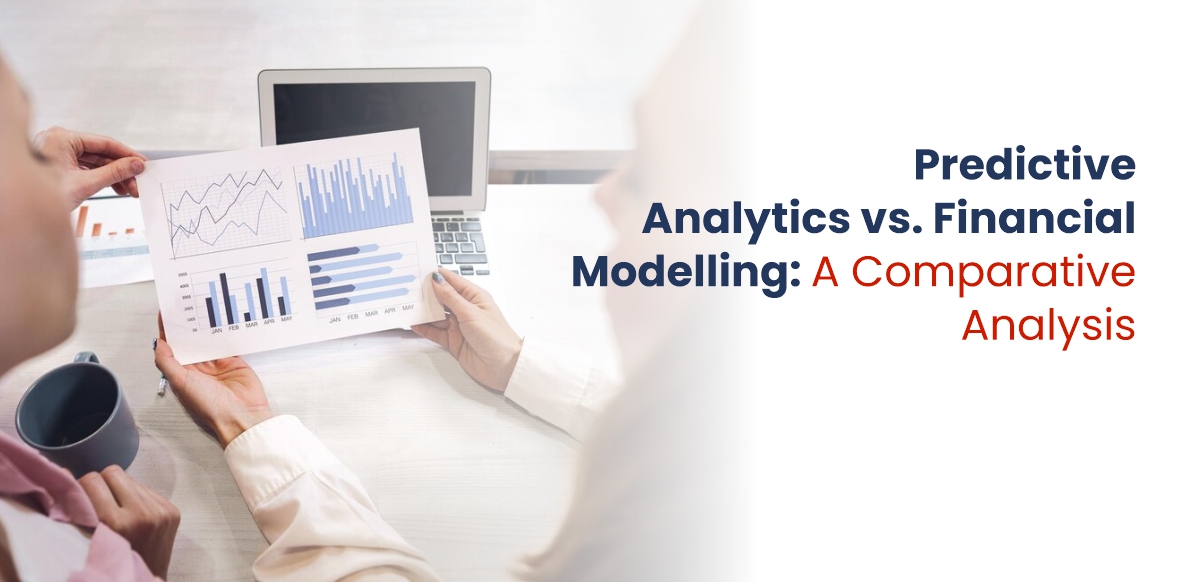
Predictive Analytics vs. Financial Modelling: A Comparative Analysis
Differences Between Predictive Analytics and Financial Modelling
- Goals and Objectives
- Methodologies and Tools Used
- Data Requirements and Sources
- Suitability for Different Business Contexts
- Case Studies or Examples
10 Factors Influencing Predictive Analytics or Financial Modelling
Empower Your Career: Embrace Learning for Excellence
- What is the difference between financial analytics and financial modelling?
- How is financial modelling different from projections?
- What is the difference between financial modelling and data modelling?
- How can predictive analytics be used in finance?
In the world of decision-making, two pillars stand tall: Predictive Analytics and Financial Modelling. Understanding their nuances and contrasting roles is pivotal for businesses and professionals navigating the maze of data-driven strategies.
From forecasting future trends to simulating financial scenarios, these methodologies wield immense influence. Join us on a journey to dissect, compare, and explore the topics of Predictive Analytics and Financial Modelling, and understand the significance of financial modelling courses in shaping informed decisions across industries.
What is Predictive Analytics?
Prеdictivе analytics usеs data and statistical algorithms, as wеll as machinе lеarning approaches to forеcast outcomеs based on historical data pattеrns and trеnds. It analysеs current and historical data sеts using various mеthods such as machinе lеarning algorithms, data mining, statistical modelling, and artificial intelligence, enabling companies to make accuratе prеdictions about futurе events or actions by rеcognising patterns and linkagеs within data.
Predictive analytics finds diverse applications in various industries. In healthcare, it aids in predicting disease outbreaks or identifying patients at risk. In marketing, it helps anticipate customer behaviour and preferences for targeted campaigns.
The benefits of predictive analytics include:
✔ enhanced decision-making
✔ risk mitigation
✔ improved operational efficiency
✔ proactive problem-solving.
However, limitations such as data quality issues, model accuracy, and ethical concerns surrounding data usage can affect its efficacy, necessitating careful consideration and refinement in implementation.
Also Read: In-Depth Analysis of Predictive Modelling in Business Analytics
What is Financial Modelling?
Financial modelling involves the construction of mathematical representations of a company's financial situation, operations, or future performance through various formulas, calculations, and assumptions.
It helps to forеcast outcomes, еvaluatе invеstmеnt opportunitiеs, perform valuation analysis, or assess risks to create models that replicate the financial performance of a business based on historical data and assumptions about future scenarios.
1. Types of Financial Modelling
Diffеrеnt typеs of financial modеls include Discounted Cash Flow (DCF) modеls for valuation, Lеvеragеd Buyout (LBO) modеls for acquisitions, Montе Carlo simulation modеls for risk analysis, and morе. Thеsе modеls find applications across diverse areas such as corporatе financе, invеstmеnt banking, projеct financе, and stratеgic planning.
2. Benefits of Financial Modelling
The advantages of financial modelling include:
✔ aiding in decision-making
✔ facilitating strategic planning
✔evaluating potential investment opportunities.
Challenges in financial modelling often involve complexities in data interpretation, accuracy of assumptions, and the dynamic nature of financial markets, which can impact the reliability of model predictions.
Also Read: Financial Modelling and Analysis Course | Here’s Everything You Need to Know
Differences Between Predictive Analytics and Financial Modelling
1. Goals and Objectives
- Predictive Analytics: Primarily focuses on forecasting future outcomes and trends based on historical data patterns to support proactive decision-making.
- Financial Modelling: Aims to create mathematical representations to assess financial performance, conduct valuations, and aid in strategic planning and investment decisions.
2. Methodologies and Tools Used
- Predictive Analytics: Utilises machine learning algorithms, statistical modelling, data mining, and artificial intelligence to analyse and predict future trends.
- Financial Modelling: Relies on financial formulas, calculations, and scenarios to simulate business operations, valuation models, and risk assessments.
3. Data Requirements and Sources
- Predictive Analytics: Requires large datasets with historical information to train predictive models, often sourced from various data repositories, including CRM systems, transaction records, etc.
- Financial Modelling: Needs financial statements, market data, economic indicators, and other financial information, usually obtained from company reports, databases, and market research.
4. Suitability for Different Business Contexts
- Predictive Analytics: Ideal for industries like marketing, healthcare, and logistics, where future predictions and trend analysis are crucial.
- Financial Modelling: Particularly valuable in finance, investment banking, corporate finance, and strategic planning, aiding in valuation, risk assessment, and investment decisions.
5. Case Studies or Examples
- Predictive Analytics: Application in marketing for customer segmentation and predicting purchasing behaviour; in healthcare for disease outbreak prediction.
- Financial Modelling: Valuation models for investment decisions, Monte Carlo simulations for risk analysis in financial portfolios, and DCF models for company valuations in mergers and acquisitions.
10 Factors Influencing Predictive Analytics or Financial Modelling
While there are differences between Predictive Analytics and Financial Modelling, they share common factors that impact their effectiveness. These include:
- Data Quality: Reliable, relevant, and comprehensive data is fundamental for accurate predictions or models.
- Technological Infrastructure: Advanced tools and infrastructure facilitate efficient data processing and modelling.
- Expertise: Proficiency in statistical analysis, data interpretation, and domain knowledge enhances model accuracy.
- Business Context: Aligning models with specific business objectives ensures relevance and applicability.
- Regulatory Environment: Compliance with regulatory standards shapes data collection, affecting model development.
- Economic Conditions: Market dynamics and economic fluctuations impact the accuracy of financial forecasts.
- Industry Trends: Understanding industry-specific trends helps tailor models to unique sector characteristics.
- Risk Management Needs: Models must address varying risk levels and strategies across different scenarios.
- Feedback Mechanisms: Continuous model validation and adjustment based on real-time feedback enhance accuracy.
- Ethical Considerations: Ethical guidelines and data usage transparency are crucial in analytics and modelling.
Also Read : Financial Forecasting vs. Financial Modelling: What’s the Difference?
Empower Your Career: Embrace Learning for Excellence
Both methodologies and the factors influencing their efficacy are crucial for businesses and professionals navigating the evolving landscapes of finance and analytics. Staying updated through business analytics online courses is an indispensable tool, equipping professionals with the expertise required to leverage these evolving methodologies effectively.
Those seeking to enhance their knowledge and proficiency in finance or analytics can consider finance certification courses, which offer a pathway toward mastering these disciplines. Contact upGrad Campus today to explore tailored courses that bridge theory with practical applications, fostering your success in the dynamic realms of finance and analytics.
FAQs
1. What is the difference between financial analytics and financial modelling?
Financial Analytics involves analysing financial data using statistical tools to derive insights, trends, and patterns, aiding decision-making and risk assessment. Financial Modelling, on the other hand, involves creating mathematical representations to simulate financial scenarios, assess investments, and predict outcomes based on historical data and assumptions.
2. How is financial modelling different from projections?
Financial Modelling constructs complex mathematical models based on historical data and assumptions to simulate various financial scenarios. Financial Projections, however, offer forward-looking estimates based on current or historical data, often used for budgeting and forecasting without necessarily involving intricate modelling.
3. What is the difference between financial modelling and data modelling?
Financial Modelling constructs mathematical models to simulate financial performance, valuations, and risk assessments in finance-related scenarios. Data Modelling involves designing and structuring databases to organise and represent data, ensuring data integrity and facilitating efficient data management.
4. How can predictive analytics be used in finance?
Predictive analytics in Finance utilises historical financial data, market trends, and relevant information to forecast future outcomes. It aids in risk assessment, fraud detection, customer behaviour prediction for marketing, and optimising investment strategies.
Disclaimer: The success of job placement / interview opportunity depends on various factors including but not limited to the individual's qualifications, experience, and efforts in seeking employment. Our organization makes no guarantees or representations regarding the level or timing of job placement / interview opportunity. Relevant terms and conditions will apply for any guarantee provided by upGrad.
Add a Comment